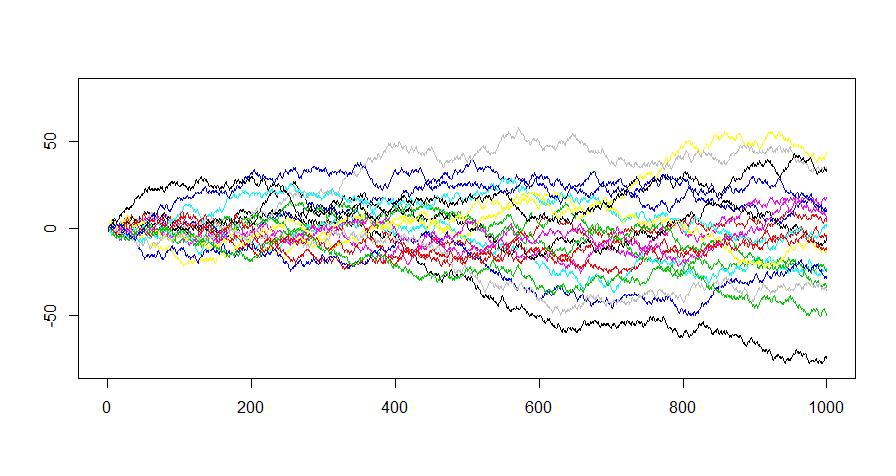
The module introduces stochastic processes, principles of actuarial modelling and time series models and analysis.
On completion of the course students should be able to (learning outcomes):
- Understand concepts of stochastic processes;
- Understand properties of Markov chain models for discrete-state processes;
- Understand applications of Poisson processes;
- Understand basic concepts to model and to analyse time series
- Understand queue models and their properties.
Syllabus:
Stochastic processes
General stochastic process models. Random walks. Reflecting and absorbing barriers. Mean recurrence time, mean time to absorption. Difference equations. Branching processes. Markov chain models for discrete-state processes. Transition matrices: 1-step and n-step. Classification of states. Equilibrium distributions for time-homogeneous chains. Detail balance, general balance, limiting distribution, stationary distribution. Poisson processes. Differential-difference equations.
Birth and death processes.
Queues. The M/M/1 queue. Differential-difference equations. Conditions for equilibrium. Equilibrium distributions of queue size and waiting time for first-come-first-served queues. Extensions to M/M/k and M/M/ queues. The M/G/1 queue, imbedded Markov chain analysis. The Pollaczek-Khintchine formula. Mean queue length and waiting time.
Time series
Time series models; trend and seasonality. Stationarity. Autocovariance, autocorrelation and partial autocorrelation functions. Correlograms. Autoregressive (AR) processes. Moving average (MA) processes. ARMA processes. ARIMA processes and Box-Jenkins methods. Forecasting and minimising expected prediction
variance. Introduction to frequency domain analysis. Spectral density function. Periodograms.
- Module Supervisor: Tolulope Fadina